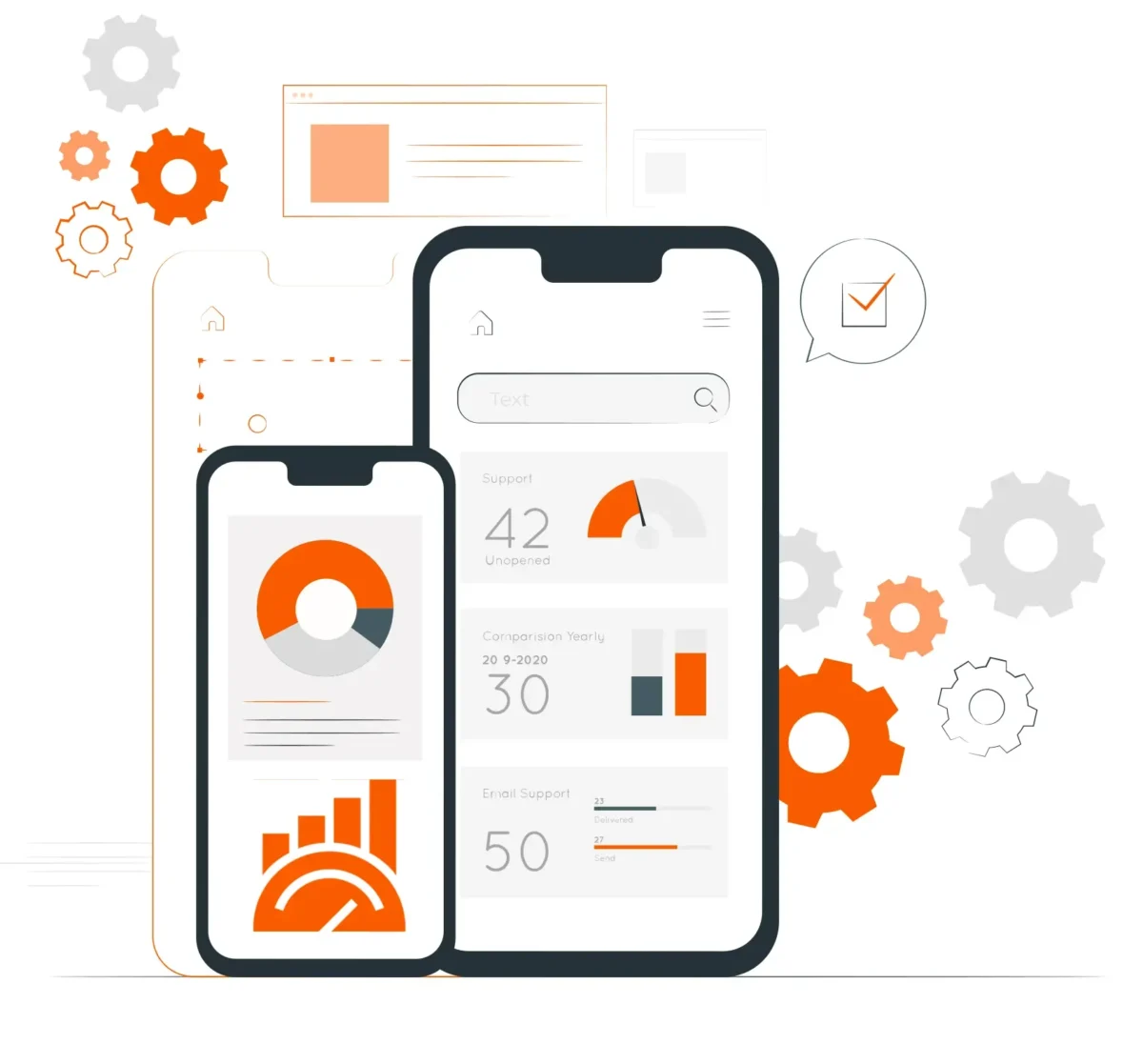
Device Performance impact on User Experience: A New Paradigm for Smartphone Benchmarking using Network Data
With the high amount of proliferation of low end / BYOD smartphones, especially in “South and Southeast Asian region”, it is imperative for a CSP to keep tab of devices & smartphone which are impacting the customer experience where network performance alone is not the limiting factor. There are bound to be Smartphones which behave differently under similar network conditions. To create a benchmark methodology where all the Smartphones in the network can be analyzed on a level scale, data collected from network and handset agents can be used.
CEMtics partnered with a Tier 1 CSP in the region to carry out an in-depth analysis based on billions of real time field samples of its network for 4G and 3G smartphones. These samples reflect the actual performance experienced by the users for various services. The analysis was carried out using data from across the nation comprising the major metros and tier 2 and tier 3 cities. The uniqueness of this big data-based analysis is that it is multi-dimensional and considers a Smartphone’s capabilities, (both software and hardware based) along with performance to arrive at a comparative scorecard. CEMtics’ s Machine Learning and Big Data Analytics capabilities were vital in enabling this analysis and building a Smartphone OEM scorecard. This helps the CSP’s in “customer experience” initiatives wherein they can ascertain impact of smartphone performance on the network and work closely with device OEMs with insights to help improve their products.
Pls note that we are not sharing any scorecard as the same is part of the contractual agreement with the CSP, as part of this blog only the approach and methodology is being communicated.
Approach
For certifying smartphones, CEMtics used subscriber event-based network activity logs (call trace data) obtained from its network infrastructure components, which contains the subscriber specific measures, and used them to create a scoring mechanism to rank and compare smartphones across KPIs.
The events which are captured in these logs comprise of different call scenarios which a subscriber encounters like a call drop, poor coverage, missed call alerts among others while making voice calls in the network.
The mechanism adopted to differentiate between the good performing and not so good performing smartphones was made comprehensive by taking a holistic approach to include important performance KPIs. The KPIs were carefully selected to capture the customer experience and provide actionable information to determine possible cause of poor performance. Statistical rigor has been applied to every aspect of this metric to ensure an unbiased evaluation. Billions of data samples were collected, and advanced statistical methods were used to create a single metric / score card which was simple and easy to understand, enabling the CSP to make an informed decision.
*The focus of this paper are the KPIs having impact on Voice experience which constitutes the of the first phase of smartphone/device benchmarking asper agreement with CSP. The data KPIs & Volte will be included in the subsequent phases.
Forward Looking Metric
The metric will continue to evolve and be finetuned. New KPIs can be easily added without changing the overall methodology. The weights can be finetuned to changing customer behavior and expectations (e.g., customer impacting like drop call, throughput kip’s can be given higher weightage and as customer demand and expect swift service continuity, higher data speeds, so the thresholds can be adjusted accordingly). The metrics can be further enhanced to gain valuable insights by correlating with customer experience and handset churn.
The Data Sources?
The network activity logs or call trace data truly meets the definition of “Big data.”
Varity & complexity: The data from a typical CSP’s network, comes in variety of file formats like asn1, xml, flat files depending of the network equipment provider (NEP) like Ericsson, Huawei and Nokia.
Volume: The data generated runs into terabytes of size for each single day and across geographical cross section of the CSP’s market
To handle the magnitude and variety of the data, CEMtics employed its expansive and robust Big Data processing mechanism to mine and build meaningful information blocks from these disparate data types.
Based on these insights the data is converted into customer experience centric KPIs like drop call rate, repeat call rate, Missed call alerts etc. These insights are generated for each smartphone model.
The Key Performance Indicators – Building block of “The Metric.”
The CSP being a customer centric brand, the benchmarking methodology needed to put the customer front and center.
To build a single “metric” that captures the customer experience (Customer centric) and at the same time gives enough information to point to the root cause (Actionable), the KPIs selection process is crucial.
The KPIs are divided into 2 main categories Customer KPIs and Network KPIs.
Customer KPIs: The goal of the customer KPIs is to capture true customer experience. For example, for a customer using voice services, the most important and frustrating event is a call drop.
1.Call Drop Rate: This KPIs needs no explanation and is by far the biggest cause of frustration for the customers. The network monitors the ongoing calls and pegs when a customers’ ongoing call is disconnected due to reasons not triggered by the user. This is mostly due to sudden drop in signal and captures the RF performance of the device).
2. Repeat Calls: We all have been in a situation while on a call, the voice call quality deteriorates to a level where the conversation is unintelligible. In this case the customer himself disconnects from the call. These events will not be pegged as a drop as the disconnect is user initiated. To capture these events, CSP has come up with a new KPI called “Repeat Calls”. The signature of these events is that after a call between Part A and B ends in a “normal disconnect”, if either party call each other within 30 seconds then the assumption is the earlier disconnect was “abnormal” and hence captured as a “repeat call’.
Each one of the ~billion calls made on the CSP network every day is evaluated for repeat calls. CEMtics’s big data scalable algorithms make this herculean task easy. This is truly a customer centric KPI that captures a whole host of issues relating to device voice quality / acoustics, sensitivity etc.
3. Missed calls: Another event that frustrates the customer are missed calls. When a user is out of coverage or for some reason is not able to access network during a specific time, then all incoming calls in the intervening time to the user will be generated as Missed Call Alert SMS notification.
This KPIs captures the RF sensitivity of the device and likely to be more common with devices with poor sensitivity that lose the network more often. It is also hypothesized that the processing power of the device also play a role in causing missed calls.
Network KPIs: The network KPIs compliment the customer KPIs by providing more insight as to the possible cause of performance degradation. The Network KPIs capture Signal Strength and Signal Quality. The purpose of the network KPIs are to account for the scenarios wherein a user might be experiencing poor signal coverage and / or poor signal quality conditions which in turn might impact one or more of the Customer KPIs.
Signal Strength KPIs
The network experience due to these KPIs can generally be gauged by the user by looking at the signal bar display on the smartphone. The count of signal bar displayed on the smartphone varies with the RSCP and RSRP values measured by the smartphone antenna.
The signal strength is measured at the antenna receiver of the smartphone and corresponds to coverage signal radiated by the nearest mobile tower to the location of smartphone user.
- RSCP (Received signal Code Power) is the signal strength received by the smartphone antenna when the user is connected to 3G network.
- RSRP (Reference Signal Received Power) is the signal strength received by the smartphone antenna when the user is connected to 4G network.
Signal Quality KPIs
The quality KPIs are a derivative of Signal strength KPIs and is vital in influencing customer network experience. In fact, different aspects of customer experience like call continuity, internet browsing speeds are dependent on signal quality KPIs.
The signal quality is also measured at smartphone antenna receiver and is not only dependent on the coverage signal radiated by nearest mobile tower to the smartphone user location but also by the signal radiated by other neighboring mobile towers near the user measured at the same antenna.
- EcNo is the signal quality value computed by the smartphone antenna when connected in 3G.
- RSRQ (Reference Signal Received Quality) is the signal quality value computed by the smartphone antenna when connected in 4G.
Inter Technology Transitions: In addition to the above, Inter Radio Access Technology (IRAT) attempts has also been used for Smartphone scoring. IRAT attempts signify the number of transitions occurring from a higher technology to a lower technology during a call.
A total of 8 individual KPIs have been chosen for benchmarking smartphones across the network, with 6 KPIs in 3G and 2 KPIs in 4G.
Aggregating the KPIs – Building “The Metric”
Having selected the building blocks i.e., KPIs, we now need to aggregate the KPIs on a per device make & model.
Due to the variability of these KPIs, we adopt slightly different methodologies for aggregation.
Drop Calls and Repeat calls are calculated as percentage failure rate over total number of calls. I.e., number of dropped calls (or repeat calls) for every device (make and model) divided by total number of calls for that device. This is a standard approach resulting in Drop Call Rate (DCR) and Repeat Call Rate (RCR) per make/model.
Missed calls on the other hand are summed at the device level and divided by the total unique IMEI’s / handsets for that make and model resulting in an aggregated KPI, “missed call per handset / user”.
The Inter technology transition (IRAT) is also calculated as a percentage. Total number of transitions divided by total number of calls.
The Network KPIs on the other hand are recorded very differently. RSCP, RSRP, RSRQ and EcNo are distributed KPI’s. They record the number of samples at various levels of signal strength and quality.
These distributed KPIs need be converted to a single metric per device.
Example case for RSCP (signal strength)
We all know that the customer experience is poor when the signal strength is low. % of samples below a certain threshold (of signal) would be a good measure of performance. The tricky part is to determine the threshold for all the distributed KPIs.
The below histogram shows the ‘RSCP values’ (in dBm) of a “device D1” in the X axis with ‘number of samples’ corresponding to each RSCP value in the Y axis.
It can be observed in the histogram that the RSCP values are distributed in range from -40 dBm to -110 dBm with majority samples concentrated around -90 dbm
It is known as per telecom network standards that an RSCP value below -100 dBm is not healthy for call experience.
A statistical method was also employed to segregate the overall device level samples into Good, Average and Poor ‘clusters’ using Unsupervised Clustering Algorithms on Hadoop machines using Spark, with data having ~150 million records per day.
Post the cluster analysis, thresholds for Poor clusters were obtained and validated with network thresholds (Cluster 4 in the above snapshot)
Percentage samples in ‘Poor cluster’ for each smartphone/device was computed. Smartphones with less percentage samples in ‘Poor cluster’ were deemed to have better performance in the KPI.
By this was a single KPI value i.e. % of samples in poor cluster for each smartphone / device per KPI was deduced and stored.
Scaling the KPIs – Creating a level playing field
As discussed in the earlier section, not all KPIs and their constituent metrics are the same. Some of the KPIs can be represented as a percentage value on a device level whereas others some are distributed and need a level of transformation to able to be evaluated for scoring.
To create a single metric or a score that would illustrate the performance of the device we need to get the various KPIs on the same unit. This is done by scoring each KPI on a scale of 1-7. This section describes this process.
The Scalar KPIs viz. Drop Call Rate, Repeat Call Rate, missed calls per user and Inter technology transition rate are computed at each device level by aggregating the numerator metric and the denominator metric for the KPI.
In the case of Distributed KPIs, namely RSCP, RSRP, RSRQ, EcNo, the KPIs are the percentage of samples in below a defined threshold.
The smartphone pH scale
Post derivation of ‘KPIs’ at device level, the KPI values were analyzed across devices to observe how the devices compare across different KPIs.
As the min and max values of the customer and network KPIs were different, scaling function was applied for each of the KPIs to bring them onto level scale.
The theoretical value of Drop Call rate or Repeat Call will vary from 0 to 100 % but the same may not be the case of MCA which is computed as ratio. Similarly, the RSCP % poor samples may not stack up with IRAT KPI.
CEMtics employed ensemble statistical scaling methods on the KPIs to convert the final Device KPI values into a range of 1 to 7.
The focus was to capture the customer experience as closely as possible.
Devices having the best KPI values where accorded a scaled value of 7 whereas the devices with worst KPI values were given a scale value of 1.
For maintaining only prominent smartphones for evaluation, only those devices which had significant user based where filtered for scaling.
Creating “One” smartphone metric
A single definitive metric gives a clear direction to the purpose of evaluating for ranking and rating purposes in addition to aiding in comparing devices across same tier, price segments, assigning ‘grades’ to manufacturer grade among others.
The purpose of Cemtics’ s device benchmarking endeavor with the CSP was to create that ‘single metric’ which can clearly help in certifying devices based on ‘many’ performance indicators.
Post obtaining scaled values for the KPIs, a final compounded score was evaluated for each smartphone by assigning suitable weights to the Individual KPIs and creating a weighted aggregate score.
Proof is in the pudding
One of the challenges of creating a ‘Smartphone performance metric” is the test of its lucidity. To demonstrate that the scoring mechanism is robust, consistent over time, independent of network conditions and is a representation of customer experience., a series of rigorous analysis was carried out to check the consistency across time, geography, and conformity to actual customer feedback.
Temporal Analysis
Hypothesis: Smartphones ranking should not vary significantly when analyzed over different intervals of time
Approach: Smartphone scores were generated Pan Nation for different time periods over 4 months.
Results: Top 20 devices were analyzed and 19 were found to be consistently in the top 20 list showing consistency of ranking.
Result: Top 100 devices were analyzed and 97 were found to be consistently in the top 100 list.
Geographical Analysis
Hypothesis: Smartphones ranking should not vary significantly when analyzed across select subset of Good & Bad clusters of Major CSP markets.
Approach: For analysis, few good performing and Bad performing clusters or zones w.r.t network performance were selected from 5 major metro cities. The Scores were generated based on few weeks’ worth of data separately for Good and Bad clusters.
Result: Top 100 devices in good clusters were analyzed and 90 were found to be consistently in the top 100 list.
Top 100 devices in Bad clusters were analyzed and 85 were found to be consistently in the top 100 list.
Customer Feedback analysis
Hypothesis: CSP users, have an option of giving a star rating to their call experience at the end of the call. This appears as part of the End of Call notification. A user having poor call experience is bound to give a less star rating and conversely the user having satisfactory or good experience is expected to give a higher star rating.
Approach: Feedback ratings based on months data were collated across top smartphones. These star ratings where then computed across smartphones using different statistical techniques like WCI, weighted average computation to generate one customer feedback score. The customer feedback score was then correlated with Smartphone compounded score using various techniques like Spearman coefficient to check if the customer feedback rating is better for smartphones with good score and vice versa.
Result: A very good positive correlation was observed between customer feedback score and the smartphone compounded score, implying better smartphone score tends to reflect in higher feedback rating.
Additional inference
After calculating weighted aggregated score based on performance KPIs, the smartphones were sorted based on score and analyzed.
The results showed a marked difference between the good performing and Bad performing handsets.
- As can be seen in the graph below, the Bottom 20 smartphones tend to have greater than 1.5X poor Drop call rate as compared to Top 20 handsets.
- The same group of smartphones also tend to have a higher percentage of samples in poor conditions as evident from the below representation.
- The RCR trend as per the box plot below also shows a lower positioned box for Top 20 handsets indicating better RCR performance and lower mean RCR for these handsets as compared to the Bottom 20 which has higher mean RCR.
Conclusion
In summary, the undeniable impact of smartphones and other mobile devices on mobile networks, particularly in the dynamic South and Southeast Asian regions, underscores the need for focused attention. The proliferation of smartphones in these areas amplifies the influence on end-user experiences, necessitating a strategic approach to address these effects.
North American CSPs conduct thorough lab tests encompassing various performance parameters, including radio functionality, voice quality, data transmission, and multimedia capabilities. In the context of South and Southeast Asian markets, the lack of a standardized testing framework (in most countries) in collaborations with the CSP’s, highlights a discrepancy that can significantly impact end users.
It’s paramount to recognize that the practice of smartphone benchmarking from CSPs perspective, should not be a one-time endeavor but a periodic and consistent practice. As technology rapidly advances and new devices continually enter the market, conducting benchmarking exercises when new devices are launched becomes essential. Moreover, such benchmarking can be extended across different KPIs & metrics as more and more information is gathered from the network using Big Data capabilities coupled with Machine Learning. These exercises serve as a proactive measure to ensure that smartphones/devices align with network capabilities and offer optimal end-user experiences.
The approach adopted by Cemtics, exemplified through insightful analyses, lays the foundation for transformative collaboration between CSPs and Smartphone/Device Original Equipment Manufacturers (OEMs). These analyses provide a conduit for meaningful discussions addressing network management intricacies. Through concerted efforts, CSPs, smartphone manufacturers and device makers can take tangible actions to elevate the end-user experience.
By Sivaram J
(CEMtics)